Better Digital Banking Through Data Analytics
Banks haven’t seen the savings expected from the transition to digital products and services. A leading consultant for some of the largest US banks shares his step-by-step plan for using smarter customer data analytics to slash costs and improve customer satisfaction.
Banks haven’t seen the savings expected from the transition to digital products and services. A leading consultant for some of the largest US banks shares his step-by-step plan for using smarter customer data analytics to slash costs and improve customer satisfaction.
Mohamed is a finance expert who has held executive internal consulting roles at JPMorgan Chase and Wells Fargo, transforming operations and saving the companies as much as $180 million yearly. He specializes in strategic planning, performance management, and process improvement initiatives.
Expertise
PREVIOUSLY AT
Digital banking is less expensive for financial institutions than maintaining brick-and-mortar locations, and legacy banks have been shrinking retail branch networks in the US for more than a decade. The COVID-19 pandemic shuttered even more in-person services and accelerated the shift toward digital banking, which lets customers access accounts, products, and services through a website or mobile app.
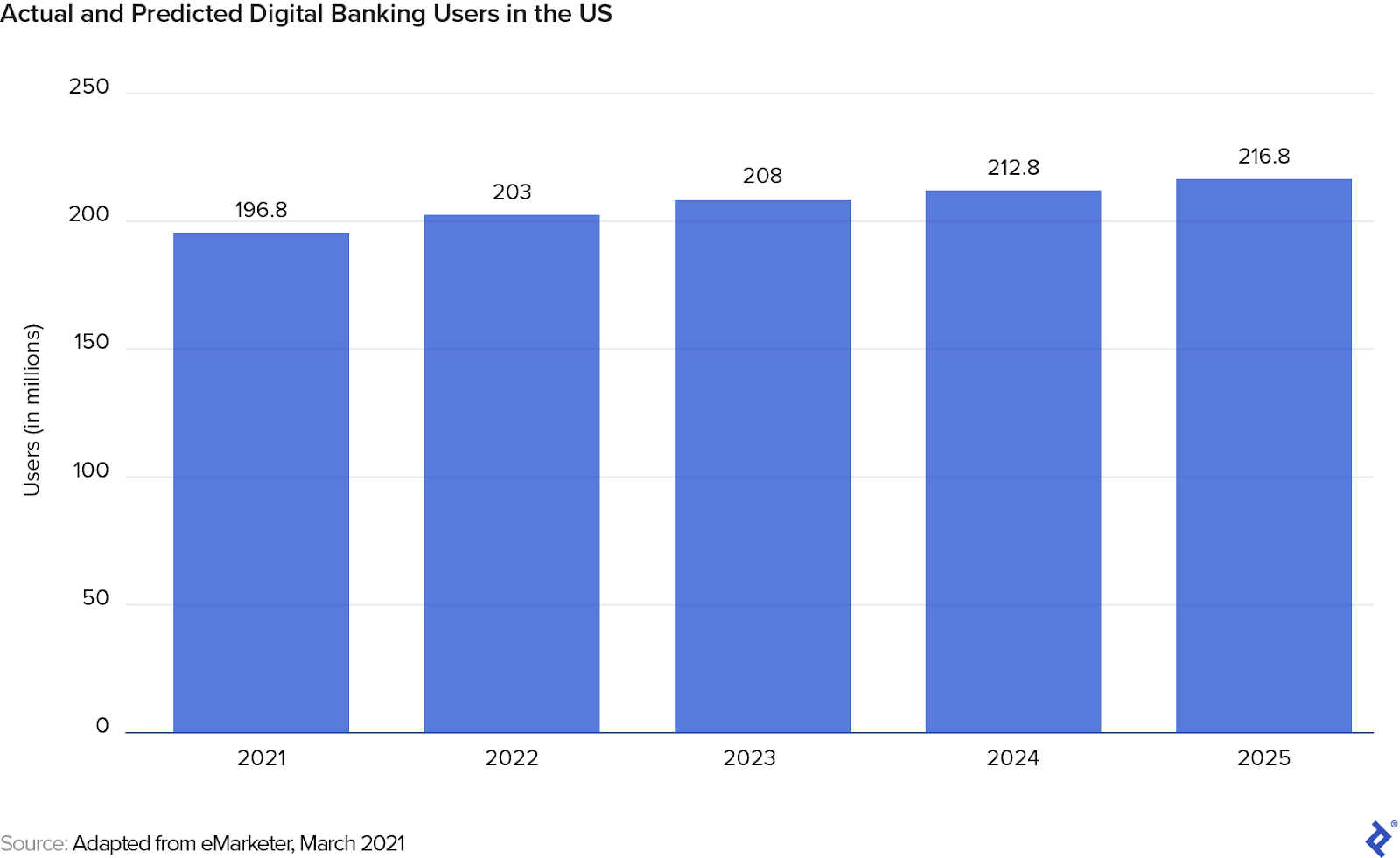
Not surprisingly, banks have been spending more than ever on technology, and improving customer experience and service delivery is their highest priority. However, financial institutions haven’t been reaping the maximum return on their digital investment because of increased spending on contact centers to deal with mounting customer concerns.
A 2020 survey from the management consulting firm Capital Performance Group found that, from the end of 2019 to December 2020, online banking activity—including transactions and other interactions—increased as much as 30% and mobile banking activity surged as much as 80%. But it also revealed that contact center volumes had as much as doubled at some institutions and continued to be elevated. And a 2021 survey conducted by Cornerstone Advisors showed that customers are calling their banks more frequently because they can’t find answers online, or their financial institutions don’t provide the virtual support needed to solve problems.
These statistics highlight the unfortunate truth that many of the digital products and services banks have been offering for years—from payment systems like Zelle to authentication—still fail to meet customer expectations, often because using them doesn’t feel intuitive enough. Even worse, Zelle in particular has been plagued by issues related to disputes, especially those concerning fraud, unauthorized transactions, and accidentally excessive fund transfers.
As a consultant at several of the largest commercial banks in the United States, I’ve seen the difficulties of digital transformation firsthand. Banks have vast troves of information that could help them develop strategies to drive customer retention and activation, but the data is underused. Most institutions’ data analytics usage isn’t nearly robust enough to capture the depth and breadth of information about customer behavior required to understand users’ needs and how best to meet them. Support teams typically use extremely small sample sizes when they’re recording and listening to calls for quality assurance—1% or less at one bank I worked with. As a consequence, extrapolating the findings can yield misleading results.
To truly address these problems, banks need to develop more detailed, holistic customer data analytics on a much larger scale—including all calls. Then they have to use the patterns of behavior they find to inform the creation and enhancement of digital functionality that satisfies customers’ needs. In this article, I’ll walk you through the steps I take to help banks do this.
Establish a Strategy Team
The first step to increase consumer adoption of digital banking and enhance customer satisfaction is to establish an in-house team composed of the bank’s analysts and product experts that will have access to data collected across all channels and products. This team is essential because there’s too much data for one individual to aggregate, study, and draw conclusions from. This group must also actively collaborate with department heads to ensure its findings can be implemented at the enterprise customer level.
I recommend that this team be subdivided into smaller cross-functional groups for each product. In my work with commercial banks, I’d tell each of these teams how many customer calls it could eliminate by implementing certain features or functions, so it could prioritize its work accordingly. To calculate this reduction potential, I used a proprietary customer journey analytics platform we’d developed to dissect the user flow and friction points. (Tealeaf and Google Analytics are similar tools that you can use for the same purpose.)
Identify Categories of Data and Set Your Goals
The second step is to determine and obtain access to the disparate sources of data across all platforms and functions. At a typical legacy bank, data sources can be broken into two categories and multiple subcategories. For example, the teams I set up at banks used these:
Products and businesses
- Retail, such as checking and savings accounts
- Credit cards
- Mortgages
- Automotive finance
- Wealth management
Points of contact
- Contact center telephone calls
- Contact center interactive voice response (IVR) communications
- In-person interactions at retail branches
- ATM interactions
- Desktop application
- Mobile application
- Outbound notifications/alerts
Much of my analytical work was delving into call statistics at contact centers, and I consider this the most important area to focus on. It’s where the overwhelming majority of support requests are made: Banks generally don’t take email inquiries for customer service anymore because it's too costly, even when done offshore. Chat has replaced email but accounts for only a small portion of all customer service interactions among top banks—less than 5% at the institutions where I consulted. Additionally, because of the high volume of requests, live agent calls are significantly costly for banks. So this is the area I’ll focus on as I explain my process.
Once data sources are identified and accessed, the financial institution can begin establishing key measurable objectives to help frame the project’s scope, which can set the stage for its problem-solving strategy. Here are the objectives we established at the banks where I consulted:
- Improve customer experience—measured by Net Promoter Score (NPS), a key indicator of customer satisfaction that assesses peoples’ likelihood of recommending a company—across all channels
- Increase digital adoption and engagement
- Reduce calls to contact centers when they aren’t adding much or any value
- Reduce low-margin, basic banking interactions at branches
- Improve efficiencies across service operations while reducing risks
As expected, the data showed that the dominant channel in terms of the volume of customer interactions is digital. Surprisingly, though, at the banks where I worked, highly active digital customers were more prone to seek support than less active digital and traditional banking users. Digital banking resulted in more than twice as many calls and inquiries into contact centers as traditional banking.
Understand Why Customers Are Calling
Once data categories and goals are identified, the team needs to consider what kinds of queries into the bank’s data will help it assess the nature and circumstances of the customer support requests. At the banks where I consulted, we focused on the contact centers, specifically information from customers’ interactions with phone agents within a given time period, and came up with the following questions:
- How many customers spoke with a live agent?
- Who were these callers, i.e., what were their profiles in terms of service interactions across channels, transactional activities across products, and customer value at the enterprise level?
- What percentage of those callers were digitally active?
- What, if any, banking activities had occurred before the call?
- If there had been a banking activity prior to the call, in which channel did it take place?
- What did the customers call about?
- Did any of them call more than once? If so, how many times?
- How long did the calls last?
- For customers who made multiple calls, how much time elapsed between them?
While banks typically do track the number of calls fielded by contact centers, they usually don’t delve into many of these ancillary statistics. This is consistent with what I’ve seen more broadly in financial services: Firms track events but do a poor job of measuring activities around events that can explain behavior and help them make improvements. Knowing that a customer spent 20 minutes attempting to resolve a dispute or activate cash-back rewards before they called would give call center employees helpful context and inform their interactions.
At the banks where I consulted, I worked with the strategy teams I built to help the institutions document each call’s purpose through a system of record. For example, we could see that one customer, whose identity was anonymized, had gone online minutes before calling and tried unsuccessfully to close their account—the experience that had prompted the call. Then we assigned each call a label denoting its purpose and a time stamp. We were also able to determine secondary and tertiary reasons for the calls by identifying events that had occurred around the primary catalyst, allowing us to map out a full picture.
We subsequently calculated a key metric known as call-to-contact spread, which we used to assess the experience of the overall population of callers. It also served as a benchmark for improving efficiency.
- Call rate: the total number of calls made, expressed as a percentage of the entire customer base
- Contact rate: the number of customers who made calls, also expressed as a percentage of all customers
- Call-to-contact spread: the call rate minus the contact rate
Our first goal was to reduce both the call rates and contact rates, because they were trending higher than the industry benchmarks for top banks, which usually run around 20% and 10%, respectively, for a given month, based on my experience and reports from third-party benchmarking firms like Finalta from McKinsey. Second, we wanted to make the two metrics equal, meaning that we had eliminated repeat calls. Once we’d accomplished that, we’d be able to say that we had achieved first call resolution, meaning consumers only needed to make one call to get their problems solved—a key benchmark in customer relationship management.
The other related metrics we analyzed and hoped to decrease included:
- Call durations
- Call transfers
- Escalations
- Complaints
Most contact centers use the call reasons acquired from customer relations management systems or call recordings, but very few use the system of record to drive improvements on these metrics. The upstream conditions in the system of record can eliminate some calls altogether and, in other cases, help match callers with the right call agents, based on their activities before the call, their customer profiles, and the levels of service needed.
Most financial institutions employ customer experience management software that surveys people after interactions, producing a Net Promoter Score. At the banks I worked with, we set (and accomplished) a goal of increasing the contact centers’ scores to 55%.
Analyze Call Patterns to Create Profiles
The next step involves analyzing call patterns using various systems of recorded data points to figure out what’s driving customers to call. The comprehensive customer-level data sets we used where I was consulting included:
- Monetary transactions, such as large point of sale (POS) fees as well as payments and reversals.
- Non-monetary transactions, such as address changes, cards being declined, and disputes.
- Channel interaction events, like phone calls, IVR communications, desktop activity, mobile activity, branch visits, ATM transactions, and outbound alerts.
- Customer profile/segmentation, such as tenure, high-value flag (indicating a high level of engagement), and number of authorized users.
- Customers’ active vs. inactive product holding, i.e., the number of products each customer has and whether they’re using them, which indicates the likelihood that they’ll interact with the bank for service. (Note: We focused on active customers to measure contact and call rates.)
From these categories, we captured some key and easily accessible data elements to better understand the following:
- Channel containment: customer propensity to stay within a channel for short periods of time, generally 15 to 20 minutes
- Channel self-service activity: what the customer did to self-serve, through digital, ATM, or IVR
- Channel mode or preference: the primary channel that the customer used
After analyzing these upstream conditions, we focused on the downstream effects by measuring and analyzing what was going on with live agent calls. Excluding calls that lasted less than 60 seconds, we examined call patterns and types, calculating time ranges, identifying how calls were made, and determining whatever drivers we could. We also collated the data so we could see the channels where customers had been banking.
After applying more advanced analytics and measuring time between calls, we grouped the population into segments:
-
Cluster callers: These were customers experiencing issues who could not self-serve, needed human help with multiple interactions, and placed a high number of calls within a short time period. (We defined this as two or more calls within 24 hours.)
-
Scattered callers: These transaction-driven callers made a lower number of calls than the cluster group, at a regular cadence and with similar types of inquiries. Scattered callers placed three or more calls across six months with an average time between calls of more than 48 hours and a coefficient of variation of average time between calls greater than 100%.
-
Random callers: This segment was composed of information-seeking customers characterized by an irregular volume of calls with different types of inquiries.
Address Callers’ Needs by Group
Once call pattern analysis is complete, it’s time to parse the data further by group. At the banks where I worked, this task revealed interesting and potentially actionable insights. At one of them, for example, the analytics showed that 5% of the population were cluster callers, 30% were scattered, and the remaining were random. The cluster population was primarily digital, while the scattered exhibited less digital activity, with a portion being habitual or heavy callers.
This table highlights a 12-month view of the live agent caller population taken at the end of the three-year buildup around this effort:
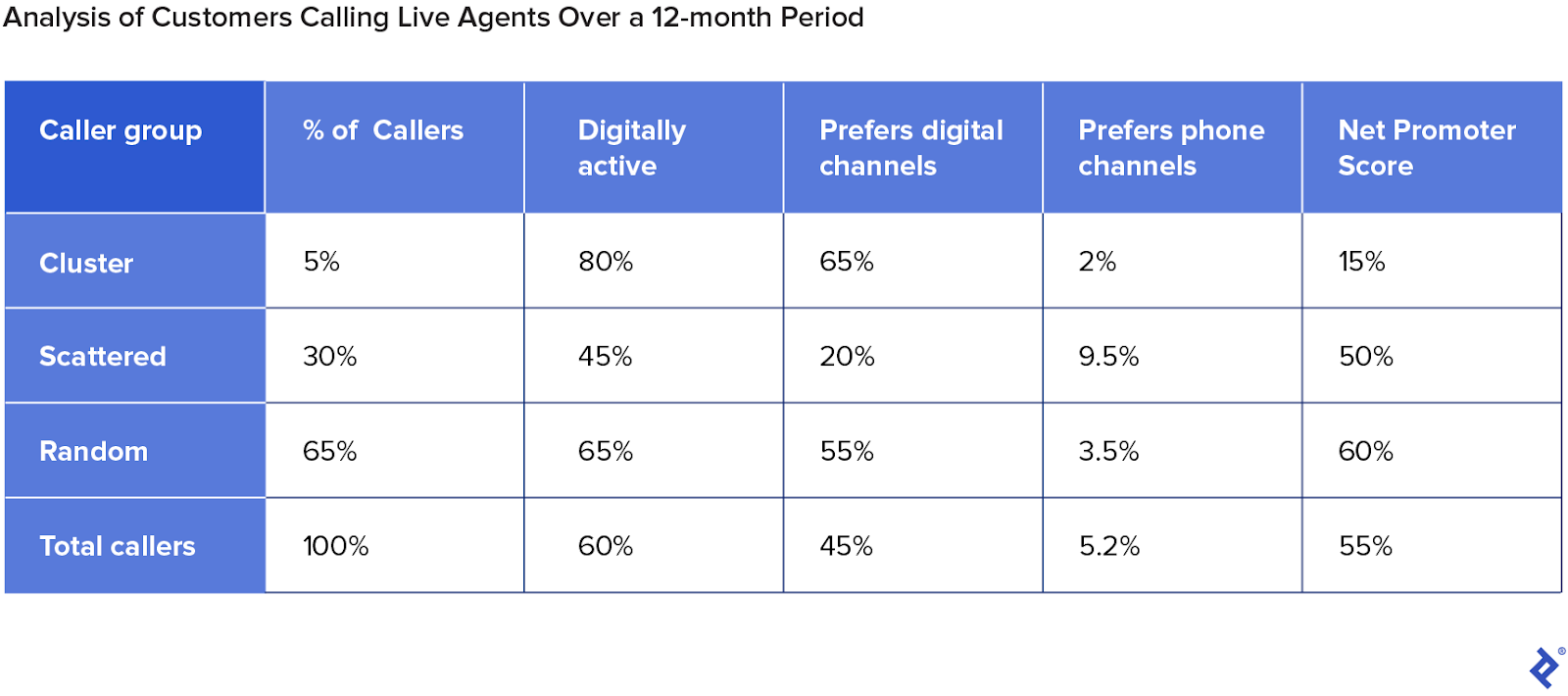
The cluster population, while small in number, has a big effect on NPS and complaints. We followed these findings by producing a series of insights with recommended strategies to meet key objectives, starting with the cluster group. Here’s a breakdown of the issues that were found to be driving calls:
- Password/username authentication
- Fraud/unauthorized transaction
- Zelle payment
- Undelivered outbound alerts
- Overdraft/insufficient funds fee
Based on our enhanced understanding of callers’ motivations, the first step we took to reduce cluster callers was to recommend specific ways to improve the online experience and contain customers within the digital channel they preferred. I also worked with the customer experience team to better design targeted surveys to assess pain points and issue resolution, and we tapped a queue of fully empowered phone agents to handle this group. These agents could waive fees or penalties, whereas others could only refer callers to more senior representatives.
For a high proportion of the cluster population, one bank had incorrect email addresses on file, which prompted a wider look that showed incorrect email addresses for more than 10% of all digitally active customers. A campaign was rolled out via push notifications and secure emails to prompt customers to confirm and change these addresses.
Scattered callers were driven more by routine services like payments, deposits, and cash withdrawals, and less by digital activities like Zelle fund transfers. Their channel preference was phone, and almost 10% of them were labeled as heavy or habitual. The strategy for this group was targeted messaging and guidance toward digital adoption and engagement.
Many random callers were inquiring about new features, products, and hours of operation for particular branches. Some of this information was added to the online experience to reduce calls. At the height of the pandemic, customers had to make appointments to go to branches. The call centers at one of the banks where I consulted were fielding thousands of calls to set these up, so I worked with the digital team to enable customers to make appointments online, eliminating many calls and saving money.
By grouping callers into three buckets, we were able to establish a call pattern effort that helped the banks I worked with better understand the drivers of behavior and how different channels were being used, especially digital versus phone. This strategy was central to cutting contact center expenses, which were increasing even as the customer base remained flat. It also aimed to improve customer experience and service levels while minimizing wait times, transfers, escalations, and callbacks. The results from one bank in particular were profound after three years.
- Digitally active rate increased by 10%
- Contact rate decreased by 12%
- Call rate decreased by 15%
- NPS increased by 5%
The drop in contact and call rates also reduced several key risk factors. In identifying calls that didn’t add value to the bank, we found that many live agent phone functions could instead be performed by the customers in self-service channels, which saved money and decreased the likelihood of human error around disclaimers, product changes, account openings and closures, and other services that customers inquired about.
There’s no shortage of data available for analytics in banking and other financial services, and reducing contact center calls and expenses is just one example of how you can use it to drive positive transformation: You may well find another focus or use a multipronged approach. The challenge is to transform your information into insights that enhance customer experience, increase digital engagement, and save money. Fully understanding what’s working—and what’s not—can ultimately lead to nuanced changes that make the system work better for everyone.
Understanding the basics
How is data analytics used in banking?
Data analytics is the study of data sets to find patterns and draw conclusions. Banks use it to assess customer engagement and satisfaction across their products and services.
What is meant by digital banking?
Digital banking refers to the use of financial services and products through a bank’s website or mobile app. It includes all kinds of bank-related activities, such as opening or closing an account, configuring alerts, disputing fraudulent transactions, and depositing, withdrawing, and transferring funds.
Why is digital banking the future?
Digital banking is theoretically less expensive for providers, and legacy banks have been slashing US retail branches for more than a decade. Properly implemented, it’s also faster and more convenient for customers. Despite its current imperfections, its use has increased year over year.
Mohamed Zarrugh
Scottsdale, AZ, United States
Member since May 12, 2022
About the author
Mohamed is a finance expert who has held executive internal consulting roles at JPMorgan Chase and Wells Fargo, transforming operations and saving the companies as much as $180 million yearly. He specializes in strategic planning, performance management, and process improvement initiatives.
Expertise
PREVIOUSLY AT